Why We’re Still Far From True Artificial General Intelligence
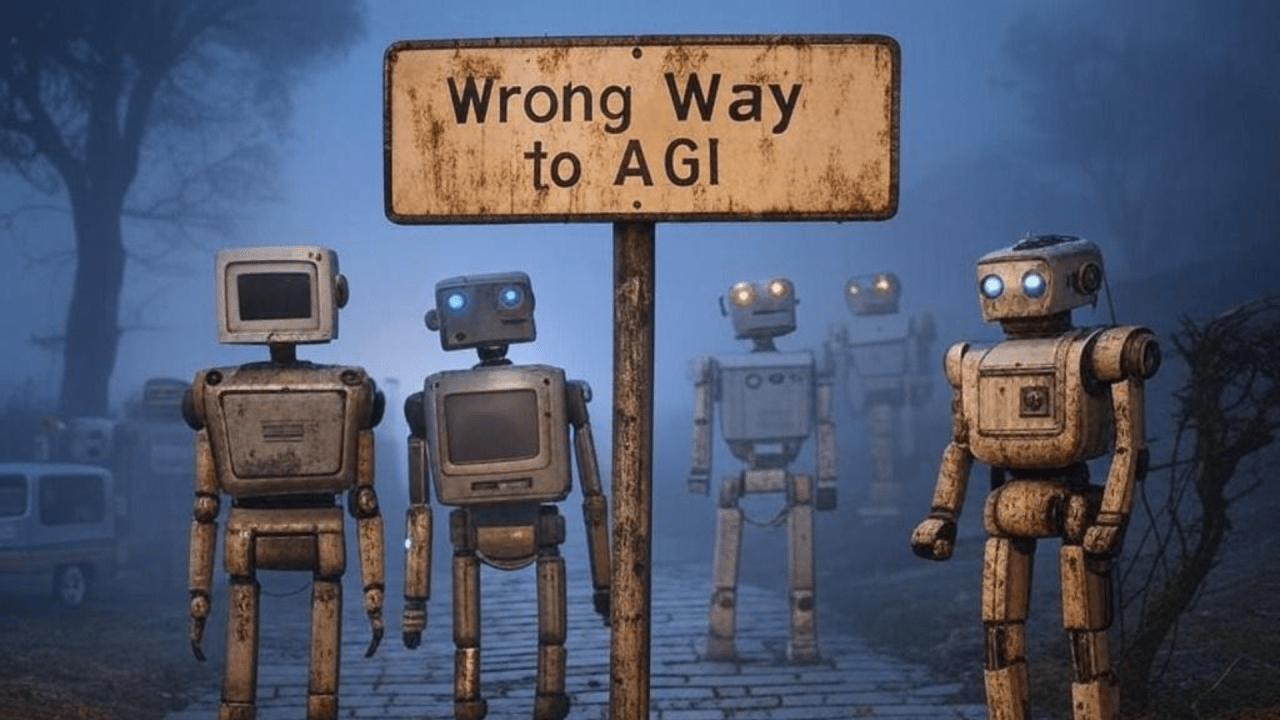
WHY THE CURRENT EFFORTS ARE NOT GOING TO TAKE US TO AGI or even ASI?
For me as someone who has spent the past 10 years around AI and automation buinding software and websites for lead generation, the advancements have been mind-boggling over the years. Whether it’s language models to write essays or vision algorithms to identify deep images, narrow AI has proven transformative.
But in the case of AGI (Artificial General Intelligence) and ASI (Artificial Superintelligence), we are still several leagues behind. Amid the hype and massive AI investments, the reality is grim: we are not getting very near to AGI, and here’s why.
1. Lack of True Understanding
Currently available AI models, such as large language models (LLMs) such as ChatGPT, excel at statistical correlations, patterns and probabilistic predictions. But they do not “know” the world like humans do. This very contrast, understanding and computation, is the reason AGI is still a mystery.
Let’s think of Google’s Bard or OpenAI’s GPT-4. These models produce persuasive prose but not proper comprehension or meaning. When you ask them a philosophical question or give them an ethical problem, they might respond well but with little thought or reason since they cannot really “think”.
My suggested Hijack: In order to close this chasm, neuroscientific advances and cognitive modeling are required. Teams have to do more than scale models, they must adopt learning models such as symbolic reasoning or causal inference (which has been sidelined in the name of speed and efficiency).
2. Overemphasis on Data and Scale
We live in a world where “bigger is better” has become the mantra of the AI community. You see it in the actions of companies like Microsoft, Nvidia, X, and Google, which are pouring billions into servers, larger datasets, bigger models, and more computational power, believing this will bring us closer to AGI. However, this methodology is running out of steam. It’s becoming increasingly clear that the focus on scale, rather than intelligence itself, is a fundamental flaw.
Take Microsoft’s recent $80 billion investment in data centers and GPUs as an example. While this infrastructure is crucial for scaling narrow AI, it does little to advance genuine intelligence. Building bigger doesn’t mean building smarter.
I can’t help but draw a parallel to the biblical story of creation: God didn’t build billions of houses before creating humanity—He created a single man. Similarly, to achieve AGI, we must focus on creating one true intelligence rather than blindly scaling infrastructure (makes no sense). It’s time to rethink our approach.
The Catch: With scaling comes a price. We’ve seen colossal machines use billions of dollars in energy and materials without equal increases in reasoning or knowledge. ChatGPT-4, for instance, although bigger and better than its predecessors, still fails at low-level reasoning that calls for causal rather than pattern-based thinking.
What’s Going to Have To Change: Rather than pursue scale, it’s time to invest in new architectures that model human logic and mimic emotions. This needs to be a smaller, smarter machine that makes sense and finds solutions (not simply parsing huge datasets). I wish I can have just $80M for my AI project and I could get closer to AGI faster than anyone.
3. Missing Multimodal Integration
Human intelligence is inherently multimodal. The more I think about AGI, the more I am forced to explore different aspects of the human brain. As humans, we are natural communicators, seamlessly combining words, images, sounds, and contexts into a unified view of the world. We can convey powerful messages through a simple expression, a hand gesture, or even silence and a tear.
AI, on the other hand, remains largely a monopolistic entity, excelling at individual modalities but struggling to integrate them effectively. If you consider the advancements in AI, it’s true we’ve made significant progress compared to 10 years ago. However, when compared to the goal of achieving AGI, we’re still far behind. AI today can create videos, generate images, clone voices, and produce text, but all of this operates under the predefined instructions of a programmer who dictates the outcomes—even if it appears to be the AI’s natural “choice.”
We are still playing a game of giving instructions, training agents, designing bots, and programming sequences and actions. What we are doing is merely anticipating outcomes and coding to predict them. The reality is that AI development is still viewed as a tool to perform tasks for us, rather than as a system that requires true freedom to evolve and think independently.
Point of Example: Take OpenAI’s effort to create GPT models for text and images, or Google’s work on visual language models. Promising though they might be, these systems cannot combine information as a human physician might; like looking at a patient’s history, imaging scans and lab results at the same time to make a diagnosis.
Why You Should Care: Multimodal integration is essential in a domain such as healthcare, where decisions are made based on mixing multiple data points. For example, an AI that can combine genetic information with past patient information and current vitals would be a game-changer in early cancer detection. Yet today we are far from this convergence.
The Road Ahead: Investments must be directed towards modality-agnostic architectures where AI becomes more of a human specialist.
4. Ethical and Safety Concerns
The spectre of AI run amok has intensified scrutiny and oversight – and rightly so. But these hurdles can slow the kind of high-risk, high-reward experiments that could spur AGI innovation.
This is an example in the real world: Driverless cars are an eye-opener. The likes of Tesla and Waymo have struggled to get their products over the regulators, despite being major advancers. AI in medicine is similarly subject to strict safety regulations, and while that’s a necessity, there’s not enough scope for radical innovation.
The Work/Play Ratio: Ethical AI design is important, but teams also need to manage innovation without safety. This, for AGI, means sandbox environments in which advanced models can be experimentally run without any harm.
5. Absence of True Collaboration
In the competitive world of AI, these kinds of ecosystems can become isolated. I think on what me and my team are doing at the moment we are working in close doors. Corporations and research labs do everything in the same way. But will be incredibly great for humanity if we can share our discoveries instead of pooling them for the good of the whole (Mankind). This incompatibility is a huge roadblock to achieve AGI in the next 5 years.
Example: Just think of the rivalry between OpenAI and Google. Both are disrupting LLMs but their own proprietary systems restrict cross-disciplinary development. How much more we might get done if their research teams came together and brought their collective talents?
The Answer to this point I’m making: In order to realize AGI, all parties must cooperate across the boundaries. Governments, corporations and academics must work together, removing silos and bringing people together. It means interdisciplinary work that crosses disciplines such as neuroscience, ethics and cognitive science.
6. Lack of a Unified Goal
The biggest hurdle to AGI might be the absence of a clear, shared objective. What qualifies as “general intelligence” varies widely across countries and organizations, leading to fragmented efforts. Some teams aim to mimic human-like thinking, while others focus on productivity and innovation in specific fields.
Then, there are the opportunists; the ones seeking to ride the hype for quick financial gains, hoping to become the next Unicorn. These are the real losers in the pool, as their short-term focus adds little to the long-term pursuit of AGI.
In contrast, there are those driven by a higher purpose: achieving breakthroughs that will transform humanity. These are the pioneers pushing advancements in health, communication, collective development, and solving the world’s most pressing problems. Their work holds the promise of redefining our future, yet the lack of alignment across the broader AI community slows progress.
To truly move the needle on AGI, we need a unified vision that prioritizes meaningful advancements over fragmented agendas and fleeting profit.
The Impact: Misallocation of resources arises from this division. OpenAI’s successes at creative text generation, for example, might be cool, but they won’t make us all AGI. Meta’s call for LLaMA models is similar: more size than substance.
What’s Needed: AI will need to reach a concrete, open-ended definition of AGI and establish a roadmap that prioritises the steps-on-the-road towards it. This means spending money on models that solve real problems and not just produce statistical output.
The Path Ahead
It’s important to understand that achieving AGI requires a shift in mindset; we need to deeply integrate the concept of AI into our core understanding, rather than treating its evolution as merely building infrastructures for mass production. The path to AGI demands a fundamentally different approach. Here’s how I see the journey unfolding:
- Defer to Substance rather than Scale: Focus more on architecture innovation and reasoning instead of model size.
- Propel Cross-Centric Research: Blend cognitive science, neuroscience and ethics to create models that “think” not predict.
- Encourage Collaboration: Disrupt silos between private firms, research institutions and governments to achieve joint development.
- Re-Define Objectives: Conclude on a common definition of AGI and identify work that is going to get us close to it.
- Don’t Invest in Causality: Build AI that can perceive and reason about cause and effect.
AGI is not just a technical problem, it’s a social one. To achieve it you need alignment, creativity and willingness to try more things. But it is not a matter of if we can create AGI but if we can make it wisely. How we deal with the creation of AGI today determines whether real AI will ever arrive.